Insights
Harnessing AI for Smarter Clinical Data Management in Biometrics
27 Nov, 20249 minsThe growing demands of Clinical Data Management require innovative approaches to ensure accu...
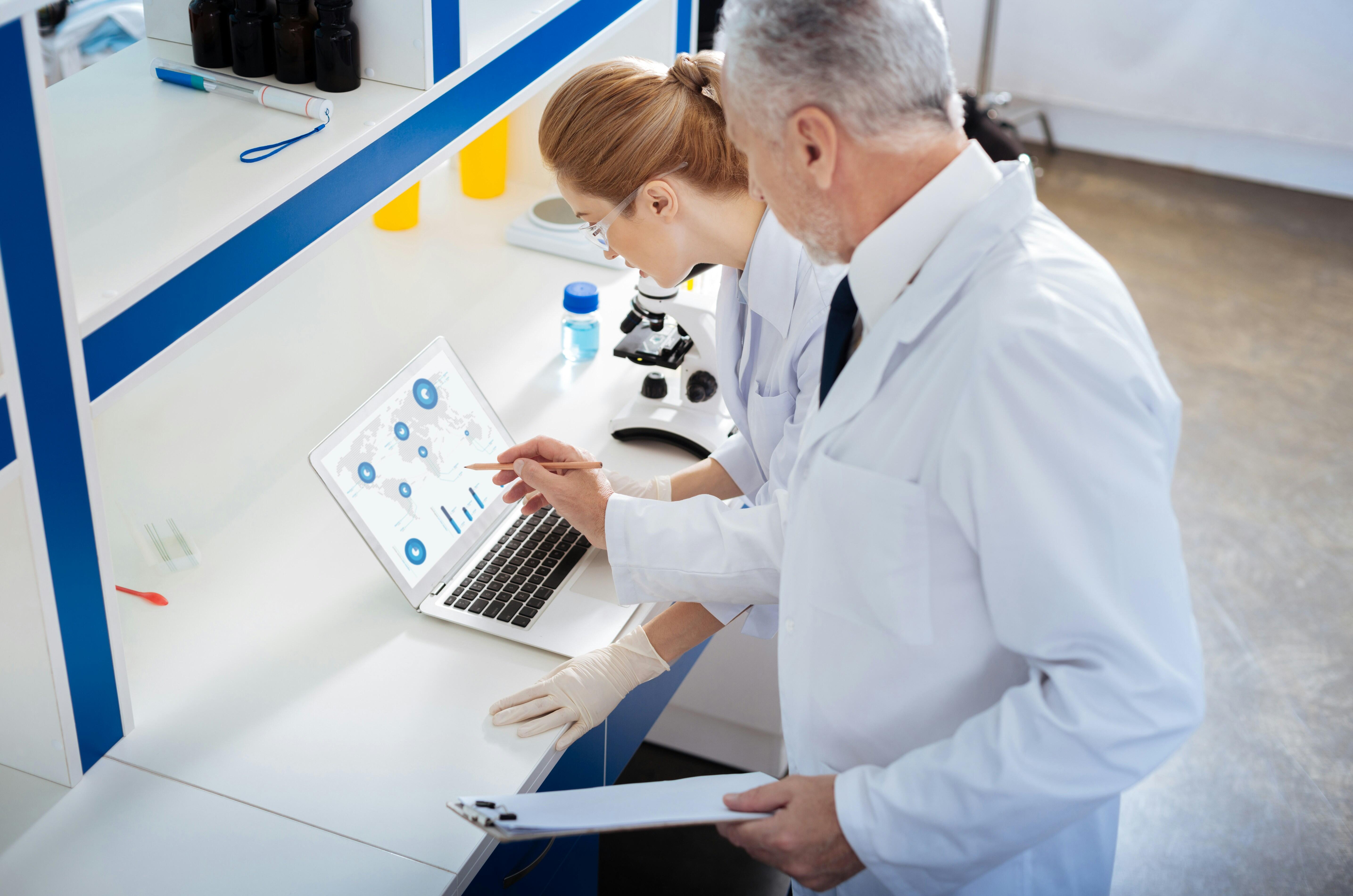
The growing demands of Clinical Data Management require innovative approaches to ensure accuracy, efficiency, and reliability. As trials become increasingly complex and Biometrics more critical, advanced technologies are complementing traditional methods to meet these challenges. AI and machine learning are playing an important role, helping to streamline processes, support data-driven decision-making, and enhance the quality of clinical outcomes.
These advancements are not only changing how data is managed but also influencing the roles involved. From integrating biometric data into workflows to improving data consistency and analysis, these technologies are shaping the future of clinical trials. Balancing innovation with the expertise of clinical teams remains central to ensuring these tools are applied effectively and responsibly.
In this guide, we’ll explore how AI and machine learning are shaping Clinical Data Management, focusing on their impact on roles, the use of biometrics, and their potential to address future data challenges.
The Current Challenges in Clinical Data Management
Clinical data management is facing increasing complexity as personalized data evolves. The introduction of biometric data and real-world evidence has brought new opportunities, but with them comes a range of challenges.
Teams must now handle larger datasets, meet stricter regulatory standards, and ensure accuracy and reliability, all within tight time constraints. These challenges are reshaping clinical trial conduct and demanding new levels of efficiency and precision.
Data Complexity and Integration
The growing volume and diversity of data in clinical trials require careful management. Biometric data, collected from wearable devices and patient monitoring, adds significant value but often arrives in inconsistent formats. Integrating this data with real-world evidence and traditional clinical datasets is time-consuming and requires specialized expertise.
For data managers, preparing this information—resolving inconsistencies, handling missing values, and ensuring quality—has become one of the most demanding aspects of their work.
At the same time, more sophisticated data analysis methods are needed to extract meaningful insights. Statistical models for advanced biometrics, such as time series or mixed-effects models, are essential but require computational resources and expert oversight. These processes must be managed carefully to avoid delays and ensure accurate and actionable results.
Regulatory and Ethical Challenges
The introduction of stricter data privacy regulations, such as HIPAA and GDPR, has added another layer of complexity to clinical trials. Protecting sensitive biometric data is essential, not just for compliance but for maintaining trust. Any misstep in data security or privacy can have serious consequences, both legally and ethically.
Beyond privacy, ethical considerations are becoming more prominent. Ensuring informed consent, addressing biases in biometric data, and clarifying ownership of patient information are critical elements in building trust with participants and stakeholders. These challenges require time and attention, further stretching the resources of clinical teams.
Operational Pressures
Existing clinical data management processes are struggling to keep up with the demands of modern trials. Data cleaning and preparation consume significant time and resources, leaving less room for higher-level analysis and decision-making. Sensitivity analysis, used to evaluate how different assumptions impact trial outcomes, is another time-intensive task that requires careful planning.
Regulatory reporting adds further pressure. Preparing detailed submissions for organizations like FDA and EMA is vital but resource-heavy. Balancing these operational needs with the ongoing demand for innovation and efficiency is one of the greatest challenges teams face today.
As the industry transforms, the need for solutions that can balance the increasing demands of complexity, compliance, and efficiency is greater than ever.
AI in Biometrics and Clinical Trials
AI is transforming clinical trials and biometric data by enabling smarter data use and reshaping processes. Its applications range from enhancing patient selection to optimizing trial designs and monitoring participants in real-time. These advancements are addressing challenges in clinical data management, ensuring efficiency, accuracy, and patient engagement while adhering to Biometric Data privacy laws.
Let’s discuss how AI influences patient selection, trial designs, and real-time monitoring while tackling ongoing challenges in Clinical Data Management.
Smarter Patient Selection and Adaptive Trial Design
AI tools are helping refine patient selection by analyzing biometric data from wearables like smartwatches and biosensors. This allows researchers to identify candidates who meet trial criteria with greater precision, ensuring protocols are tailored to individual needs. Predictive models also enable researchers to anticipate how participants might respond to treatments, improving safety and outcomes while moving away from rigid, standardized designs.
Adaptive trial protocols, powered by AI analytics tools, adjust dynamically based on real-time insights. These adjustments ensure trials stay relevant and practical, addressing unexpected trends or challenges as they arise.
Real-Time Monitoring and Decentralised Trials
Wearable devices such as biosensors and smartwatches are revolutionizing Clinical Data Management by enabling continuous data collection without frequent site visits. AI processes real-time data, such as heart rate or blood pressure, to quickly identify trends or anomalies. This supports decentralized trials, reducing patient burden and improving engagement while maintaining oversight.
Decentralized trials are also more inclusive, allowing patients who cannot frequently travel to participate remotely. Combined with AI’s ability to integrate biometric data securely and analyze it efficiently, these approaches are helping trials become more accessible and scalable.
Broader Applications of AI
AI is transforming clinical trials by addressing challenges that once slowed progress and strained resources. Its expanding role includes:
- Risk-Based Monitoring: Early identification of anomalies in trial data allows researchers to address potential issues before they escalate, improving consistency and accuracy throughout the trial process.
- Enhanced Retention: AI analyses participant engagement patterns and predicts dropout risks, enabling timely interventions that reduce delays and maintain robust datasets.
- Personalized Medicine: By identifying biomarkers and genetic variations, AI supports customized treatment plans, ensuring better outcomes and greater participant safety.
- Regulatory Compliance: AI simplifies adherence to frameworks like HIPAA and GDPR by securely handling biometric data and streamlining compliance processes.
Several organizations are already using these advancements to drive innovation in clinical trials:
- AstraZeneca: Applying generative AI to identify drug targets and explore oncology treatments, refining trial designs, and accelerating research.
- Amgen: Analysing large-scale biometric and genetic datasets with AI, speeding up early-stage drug discovery and improving candidate selection.
- Owkin: Enhancing trial designs by optimizing patient subgroups, endpoints, and treatment effects through advanced AI tools.
These examples show how AI is helping research teams tackle persistent challenges while creating more efficient and patient-centered trials. By integrating AI into their processes, organizations are building a foundation for more personalized, accurate, and innovative approaches to clinical research.
Ethical Considerations and Opportunities
The integration of AI in Clinical Data Management presents both exciting opportunities and pressing challenges. These technologies have the potential to revolutionize drug discovery, patient recruitment, and trial design, but their implementation raises concerns about data security, privacy, and fairness.
Striking the right balance between innovation and responsibility is key to maximizing the benefits while addressing the risks.
AI brings opportunities to streamline clinical research processes and improve patient outcomes:
- Accelerated Drug Discovery: By analyzing extensive datasets, AI can identify potential drug candidates quickly, shortening the development timeline.
- Personalized Medicine: AI enables tailored treatments by analyzing real-time patient data, leading to safer and more effective therapies.
- Enhanced Patient Recruitment: Sophisticated algorithms match patients to trials based on symptoms, data, and characteristics, improving diversity and reducing recruitment delays.
- Adaptive Trial Design: AI-driven protocols adjust in real-time, ensuring trials respond dynamically to emerging data trends.
- Real-Time Data Analytics: Wearable devices continuously collect data, allowing researchers to identify anomalies or trends as they occur.
However, the adoption of AI also presents risks and challenges that demand careful attention:
- Data Privacy and Security: The use of sensitive biometric data must comply with strict regulations like HIPAA and GDPR, while fragmented state laws in the US add complexity.
- Ethical Concerns: Questions about informed consent, data ownership, and the potential misuse of patient information require robust oversight.
- Algorithmic Bias: Flaws in training datasets can lead to biased results, undermining the accuracy and fairness of trial outcomes.
- Operational Challenges: AI systems require significant integration effort, from gathering high-quality data to tailoring algorithms for specific clinical needs.
While AI excels at analyzing data and increasing efficiency, it is no substitute for human creativity and judgment. Addressing these risks with transparency and clear ethical standards will ensure that AI remains a powerful and trusted tool in Clinical Research.
AI’s Impact on Roles in Biometrics
AI is reshaping roles in Biometrics by automating repetitive tasks and changing the focus of critical responsibilities. It’s not about replacing professionals but equipping them with tools to work more efficiently and strategically. New skills are emerging as essential, blending technical knowledge with ethical oversight to ensure that AI systems are used effectively.
Shifts in Key Roles
AI has streamlined previously time-intensive tasks, such as data cleaning, validation, and monitoring. For Clinical Data Managers (CDMs), this means:
- Focusing on data interpretation and trial strategies rather than routine manual processes.
- Leveraging real-time insights to address trial risks and improve outcomes.
- Gaining expertise in machine learning, programming, and data science to guide AI tools.
Biostatisticians are also seeing changes in their roles:
- Advanced statistical tools powered by AI allow for deeper analysis of patient outcomes.
- Predictive analytics help refine trial designs and anticipate patient responses.
- Collaborating with data scientists is now a core part of the role, ensuring AI-driven insights are applied effectively.
Demand for Hybrid Skills
AI’s growing role has created a demand for professionals who can work across technical and traditional domains. Skills in programming, data science, and machine learning are becoming essential for both CDMs and biostatisticians.
New roles, such as specialists in machine learning applications for clinical trials, are also emerging. These professionals work closely with existing teams, ensuring that AI is implemented responsibly and data analysis supports trial objectives.
Keeping Human Expertise Central
AI tools are powerful but lack the judgment, creativity, and ethics needed to guide clinical decisions. Human oversight ensures that:
- Data is interpreted accurately, accounting for nuances that AI might miss.
- Algorithmic bias is identified and corrected before it affects trial outcomes.
- Patient trust is maintained through ethical data management and transparency.
Organizations must invest in training and create scalable strategies that integrate AI without sidelining human expertise. By balancing innovation with professional insight, teams can ensure AI enhances the work of biometrics professionals rather than overshadowing it.
Final Thoughts: AI and the Future of Clinical Data Management
AI is opening new possibilities in Clinical Data Management, especially in Biometrics. The increasing reliance on data from wearables and sensors requires professionals who can manage the technology and the ethical and regulatory considerations it raises. Balancing privacy, accuracy, and usability is a challenge that demands skilled human oversight.
This shift isn’t about replacing roles but evolving them. The growing complexity of Biometric Data calls for professionals who can navigate its nuances, ensuring meaningful insights, ethical decisions, and seamless compliance. As these changes unfold, the focus remains on using data to improve clinical outcomes without compromising trust or integrity.
For teams navigating these changes, success lies in pairing technological innovation with a strong commitment to human expertise. This balance will define the future of biometrics and its role in clinical trials.
Looking for Exceptional Talent in Biometrics?
At Warman O’Brien, we specialize in connecting leading organizations with skilled professionals in clinical data management, biostatistics, and statistical programming. With a proven track record and industry expertise, we’ve built networks across Pharma, Biotech, and CROs to meet your hiring needs. Whether you need contract or permanent placements, our tailored approach ensures the right fit for every role.
Contact us today to discover how our team can help you build the expertise your organization needs to thrive in this rapidly evolving field.